
Thus, selection bias seems to be a minor problem in this typical PK covariate analysis. Results from other estimation methods namely, first order conditional estimation (FOCE) and FOCE with interaction are also provided in the end for comparison. Analysis in NONMEM® was performed by using a first order (FO) estimation method. The predictive performances of the stepwise models were also reasonably good. which is a one-compartment i.v bolus model based on preliminary data analysis, is given below. There was a tendency to selection bias in the estimates, although this was small relative to the overall variability in the estimates. Therefore, the choice of model-building procedure in these examples could be based on other aspects such as analyst- and computer-time efficiency. Comparison of fungizo, Amphotec, AmBisome and. Ambisome (liposomal amphotericin B): a comparative analysis. The covariate models were CL (litres/h) -(0,44-00152 is WTA-21) and V1. No major differences in the resulting covariate models were seen, and the predictive performances overlapped. Nonmem users guide part v Thank you for your participation. The model-building procedures performed similarly in the data sets explored. One- and two-compartment pharmacokinetic models were compared. Information on covariates, in addition to sex, race, age, weight and height, such as concomitant diseases, co-medi- cation, smoking habits, should be collected. The population analysis was undertaken using the first-order conditional estimation (FOCE) method with interaction. 3.It is based on the principle that the individual pharmacokinetic parameters of a patient population arise from a distribution that can be described by the population mean and the interindividual variance. Pharmacokinetic analysis was carried out using the nonlinear, mixed-effects modeling program NONMEM (version V level 1.1 GloboMax LLC, Hanover, MD, USA).
#Nonmem covariate analysis software#
The bias in the estimated coefficients (selection bias) was assessed. The non-linear mixed-effects method is depicted in Figure 10.4 and is described using the conventions of the NONMEM software 2, 3 and the description by Vozeh et al. The final covariate models were compared, including their ability to predict a separate data set or their performance in cross-validation. Data were assumed to follow a log normal distribution. No covariate was applied while the covariance implemented the default diagonal pattern. Different versions of these procedures were tried (eg, treating different study occasions as separate individuals in the GAM, or fixing a part of the parameters when the NONMEM procedure was used). In NONMEM, analyses were performed using the provided one compartment oral model (ADVAN 2) with Ka, Kel and Vd parameters provided in NONMEM PK library. Using simulated and real PK data, covariate models were built applying (1) stepwise generalized additive models (GAM) for identifying potential covariates, followed by backward elimination in the computer program NONMEM, and (2) stepwise forward inclusion and backward elimination in NONMEM.

In addition, the effects of stepwise regression on the estimated covariate coefficients were assessed. It can run on all major operating systems (Windows, OS X, and Linux).The aim of this study was to compare 2 stepwise covariate model-building strategies, frequently used in the analysis of pharmacokinetic-pharmacodynamic (PK-PD) data using nonlinear mixed-effects models, with respect to included covariates and predictive performance. Pirana works with R speaks NLME, NONMEM, R, Perl Speaks NONMEM (PSN), and Monolix.
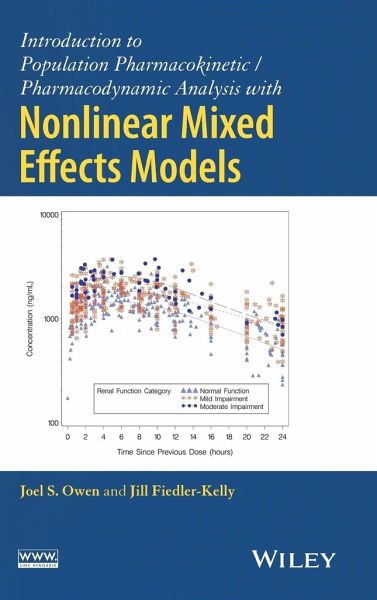

Hence, the objective of this work was to implement the Cox PH model in NONMEM® 3. A covariate model based on body weight and age and a power function model based on body weight were identified as appropriate models to describe the variability in fexofenadine oral clearance and peak concentration, respectively. Organizing and managing pharmacometric models can be an inefficient and time-consuming process. the battery of survival models to be tested in NONMEM and to be used within other procedures such as scm or randtes t in PsN 2. The data were pooled and analysed using NONMEM software, version 5.0.
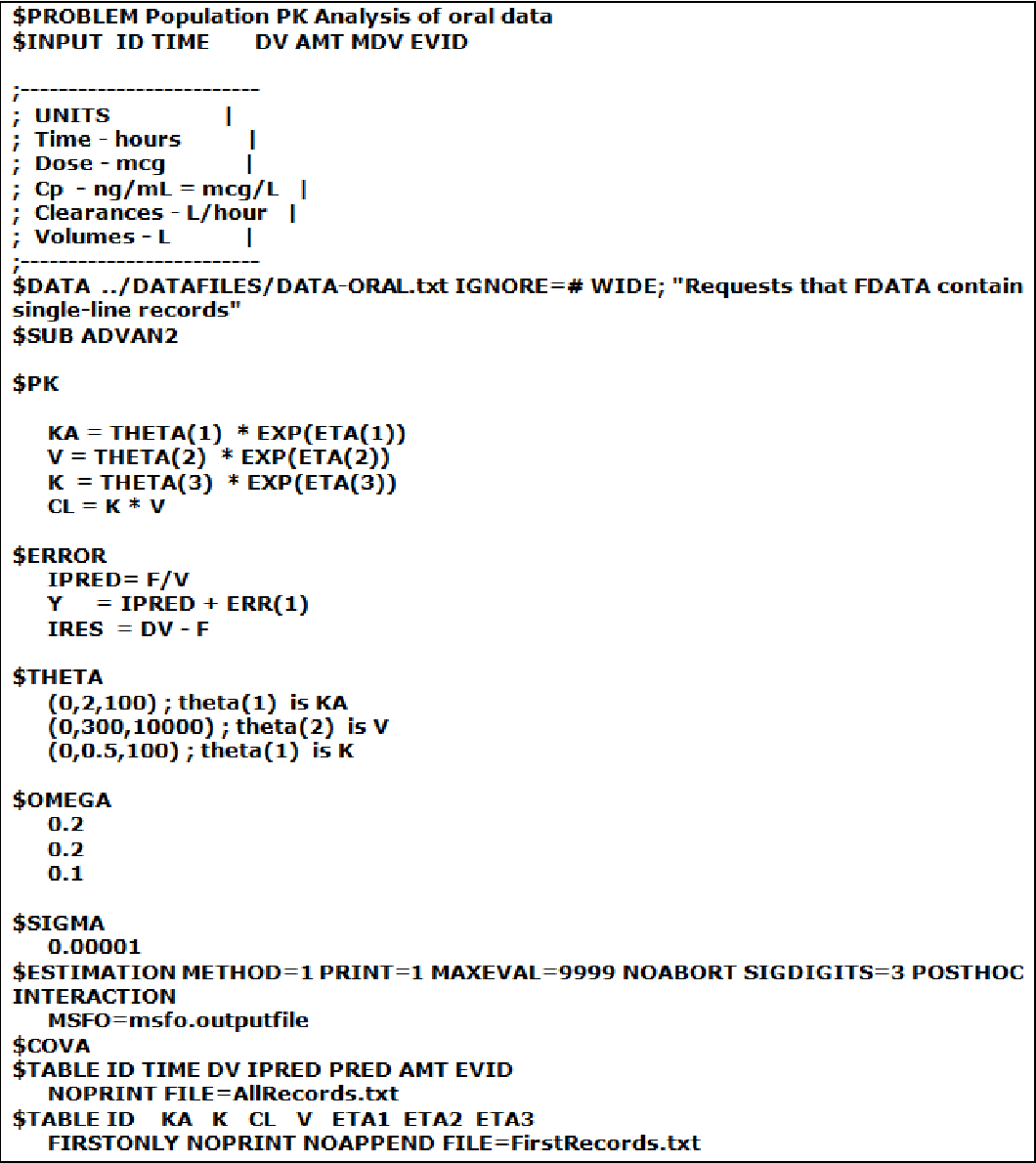
Pirana now supports R speaks NLME allowing users to run models using Certara’s NLME engine and PML syntax.Pirana Modeling Workbench Version 3.0 NEW
